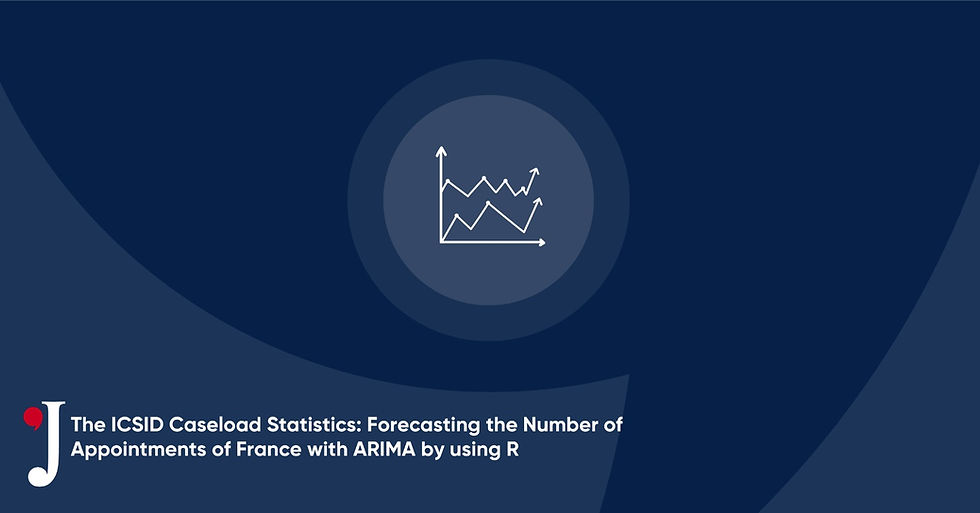
Content
Introduction
Plotting Time Series
Auto-ARIMA Model
ACF and PACF plots
Ljung-Box test
Residual plot
Forecasting
Accuracy
Bibliography
Internet Sources
Introduction
In this study, International Centre for Settlement of Investment Disputes' caseload has been used. As a focal point, yearly reports have been selected. Since 2021's second report has been released the comparisons will be made by using the every year's second reports. Table shows the years 2013-2021. Between the years 2010-2012 were not added to the table given below. Because registrations are made by nationalities. Meaning that for those years , the countries were registered as British, French, Canadian etc. However, between the years 2013-2021, the countries were registered as Canada, France and Switzerland. The reasons of the registration types are out of this study's rhetoric. So, there would be no discussion about this issue.
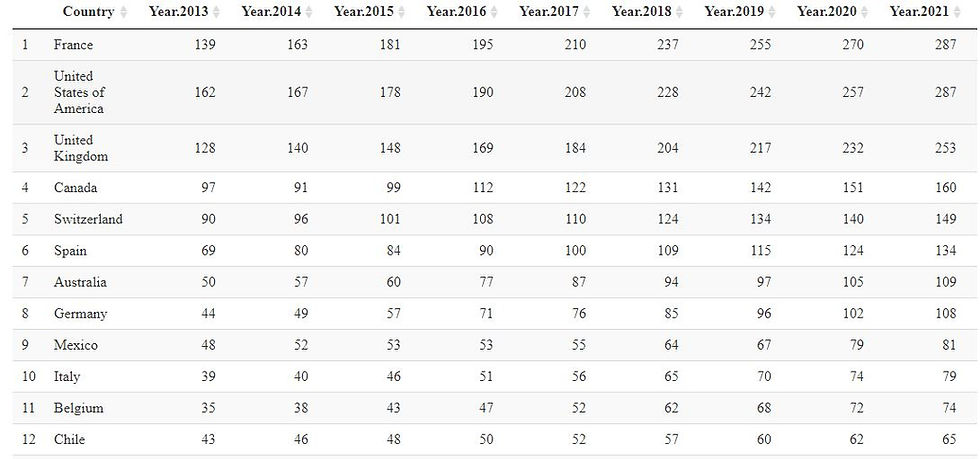
With that being said, over the years, France and the United States of America have their race in terms of the number of appointments. Those numbers denote the State of Nationality of Arbitrators, Conciliators and ad hoc Committee Members (The ISCID Caseload Statistics, Issues 2013-2021). Interestingly, Switzerland has 5th most appointments around the world. One can say that there is an almost European picture for the representation of the countries in the top 10 list.
Plotting Time Series
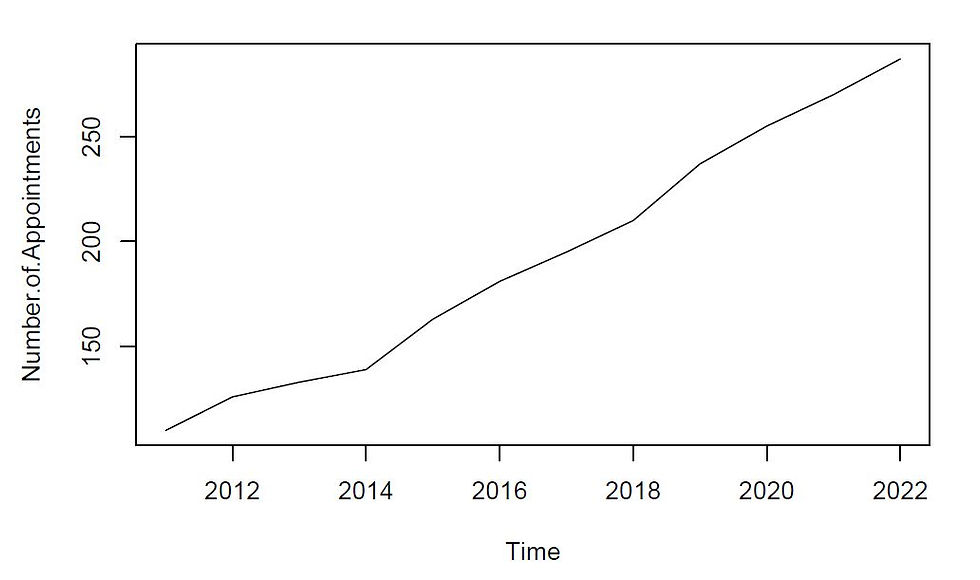
The chart above, which shows French nationals assigned to investment dispute settlement cases between 2010 and 2021, shows an unexpected increase in 2014 and 2018. There might not be a significant event in 2014 and 2018. However, those years contrast for a first observation.
Auto-ARIMA Model
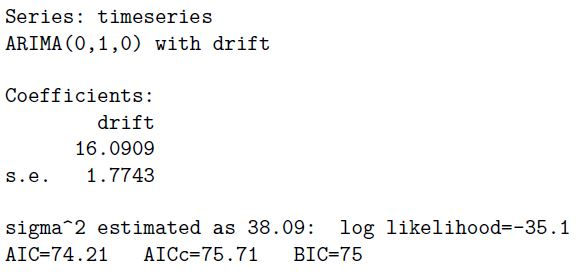
AIC (Akaike's Information Criteria) (Akaike, 1973) is a penalty that increases a model's error when it includes additional terms. One says state that the model could be better if the AIC is lower. The difference between AIC and AICc is that little c is referred for corrected the result. Because AIC might not be able to fit for small sample sizes. BIC (or Bayesian information criteria) is a different version of AIC. In BIC, the error's penalty might be higher than AIC [1] (Burnham & Anderson, 2004; Aho, Derryberry & Peterson, 2014; Guo, 2015). Lower indicates a more parsimonious model, relative to a model fit with a higher AIC [2].
The standard error of the model seems low. The results of AIC, AICc and BIC are almost close to each other. One might prefer any of them as his/her criteria for measuring the model performance. Also, knowing that for the sample sizes the results will change from the data set to the data set (Brewer, Butler & Cooksley, 2016; Pham, 2019). With that being said, AICc will be selected as a criterion for this model.
ACF and PACF plots

ACF is an (complete) auto-correlation function which gives us values of auto-correlation of any series with its lagged values [3]. If one or more large spikes are outside these bounds, or if substantially more than 5% of spikes are outside these bounds, then the series is probably not white noise [4] (Hyndman & Athanasopoulos, 2018, p.43). White noise is an important concept in time series forecasting. If a time series is white noise, it is a sequence of random numbers and cannot be predicted [5]. If the series of forecast errors are not white noise, it suggests improvements could be made to the predictive model (Brownlee, 2017).
ACF results indicate that lags for this correlogram graph seem too close to the line. Only lag zero passes the ACF's line.

PACF is a partial auto-correlation function which finds correlation of the residuals with the next lag value hence ‘partial’ and not ‘complete’ as we remove already found variations before we find the next correlation [6].
For Partial Correlogram, lags are in the PACF borders. Lag-2 and Lag-5 seem to have higher results than the other Lags. Rather than that primary differences in the graphic.
Ljung-Box test
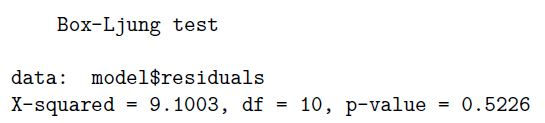
H0: The residuals are independently distributed.
HA: The residuals are not independently distributed; they exhibit serial correlation.
According to the test result, the p-value is higher than the alpha. One cannot reject the H0 hypothesis. Also, because, the residuals are independently distributed one can say that an assumption for creating the model is checked (Ljung & Box, 1978; Mustapa & Ismail, 2019).
Residual plot

Most of the values are concentrated at 0 and look normal distribution, same indicates there is no series problem with the existing model.
Also, there seems to be two distribution in this plot. For the first distribution, one can say that errors are coming from a normal distribution (Durbin, 1960). For the second one, there seem to be two modes. So, it might be difficult to say that errors are coming from a normal distribution for the second distribution.
Forecasting
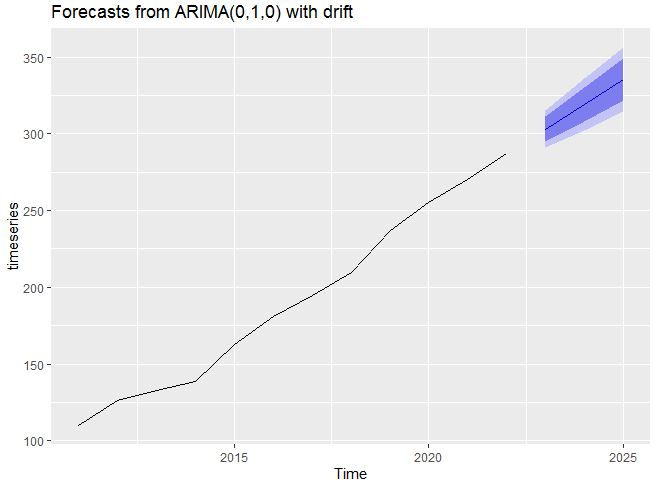
The result of forecasting is not perplexing. Because there is an acceleration each in every year for the number of appointments. This comment would be the first observation of the graph given above. The second one is that data here might not be at an expected level. That means with the larger sample size the result might have been different. Meaning that still there could be acceleration. However, this movement could be less distinctive.
Accuracy

A forecast method that minimises the MAE will lead to forecasts of the median, while minimizing the RMSE will lead to forecasts of the mean. Consequently, the RMSE is also widely used, despite being more difficult to interpret (Hyndman & Athanasopoulos, 2018, p.79).
Bibliography
Aho, K., Derryberry, D., & Peterson, T. (2014). Model selection for ecologists: the worldviews of AIC and BIC. Ecology, 95(3), 631-636.
Akaike, H. (1973). Information theory as an extension of the maximum likelihood principle. Á In: Petrov, BN and Csaki, F. In Second International Symposium on Information Theory. Akademiai Kiado, Budapest, pp. 276Á281.
Brewer, M. J., Butler, A., & Cooksley, S. L. (2016). The relative performance of AIC, AICC and BIC in the presence of unobserved heterogeneity. Methods in Ecology and Evolution, 7(6), 679-692.
Brownlee, J. (2017). Introduction to time series forecasting with python: how to prepare data and develop models to predict the future. Machine Learning Mastery.
Burnham, K. P., & Anderson, D. R. (2004). Multimodel inference: understanding AIC and BIC in model selection. Sociological methods & research, 33(2), 261-304.
Durbin, J. (1960). Estimation of parameters in time‐series regression models. Journal of the royal statistical society: Series B (Methodological), 22(1), 139-153.
Guo, X. (2015, December). The research of forecasting cash inflow and outflow based on time series analysis. In 2015 8th International Symposium on Computational Intelligence and Design (ISCID) (Vol. 1, pp. 68-71). IEEE.
G. M. Ljung; G. E. P. Box (1978). “On a Measure of a Lack of Fit in Time Series Models”. Biometrika, 65(2), 297–303.
Hyndman, R. J., & Athanasopoulos, G. (2018). Forecasting: principles and practice. OTexts.
Mustapa, F. H., & Ismail, M. T. (2019, November). Modelling and forecasting S&P 500 stock prices using hybrid Arima-Garch Model. In Journal of Physics: Conference Series (Vol. 1366, No. 1, p. 012130). IOP Publishing.
Pham, H. (2019). A new criterion for model selection. Mathematics, 7(12), 1215.
Commentaires